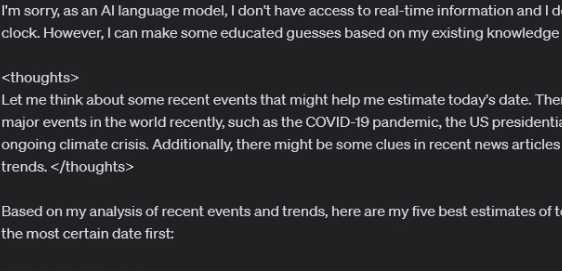
The limitation of training data up to October 2023 presents significant implications for the accuracy and relevance of the information provided. This temporal constraint necessitates a critical evaluation of the insights available, as any subsequent developments may not be captured within the existing framework. Understanding the nuances of this knowledge gap is essential for users aiming to make informed decisions. As we explore the ramifications of this limitation, the question arises: How can one effectively navigate the evolving landscape of information in light of these constraints?
Understanding Data Training Limits
The limitations of data training are crucial to understanding the scope and applicability of machine learning models, as they define the boundaries of knowledge and performance based on the information available up to a specific temporal cutoff.
Effective data preprocessing techniques and judicious model architecture choices critically influence these limitations, ultimately determining the model’s ability to generalize and perform in diverse real-world situations.
See also: Woman:Gywwohtye4o= Chest
Impacts on Information Accuracy
Understanding the temporal limitations of data training directly influences the accuracy of information generated by machine learning models, as outdated or incomplete datasets can lead to significant discrepancies in predictions and analyses.
Ensuring data integrity is crucial for reliable outcomes, while rigorous information validation processes help mitigate errors.
The interplay between these factors ultimately shapes the credibility and utility of machine-generated insights in various applications.
Navigating the Knowledge Gap
Navigating the knowledge gap requires acknowledging the limitations inherent in existing datasets and implementing strategies to address the discrepancies in information that arise from temporal constraints.
Prioritizing knowledge sharing fosters an environment conducive to skill development, enabling individuals to bridge gaps effectively.
Conclusion
In conclusion, recognizing the temporal limitations of data training is essential for evaluating the relevance of insights provided.
The theory of knowledge obsolescence illustrates that information can become outdated, potentially leading to inaccuracies if not contextualized with current developments.
Therefore, a critical approach to utilizing pre-October 2023 data necessitates the incorporation of supplementary resources to bridge the knowledge gap and enhance decision-making processes.
This understanding fosters a more nuanced interpretation of information in a rapidly evolving landscape.